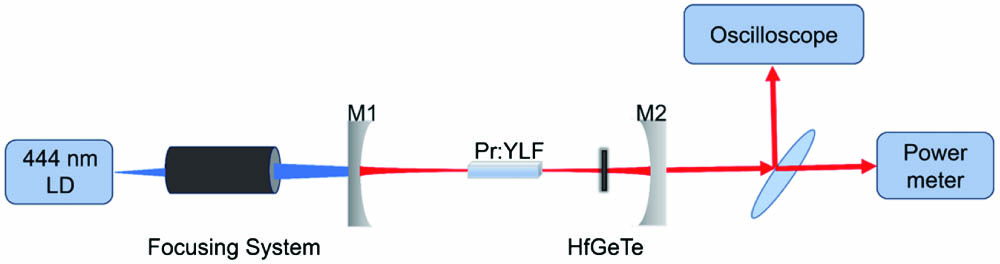
Author Affiliations
Abstract
1 State Key Laboratory of Crystal Materials and Institute of Crystal Materials, Shandong University, Jinan 250100, China
2 Beijing National Laboratory for Condensed Matter Physics, Institute of Physics, Chinese Academy of Sciences, Beijing 100190, China
Topological nodal-line semimetals attract growing research attention in the photonic and optoelectronic fields due to their unique topological energy-level bands and fascinating nonlinear optical responses. Here, to the best of our knowledge, we first report the saturable absorption property of topological nodal-line semimetal HfGeTe and the related pulse modulation in passively Q-switched visible lasers. Few-layer HfGeTe demonstrates outstanding saturable absorption properties in the visible-light band, yielding the saturation intensities of 7.88, 12.66, and 6.64 µJ/cm2 at 515, 640, and 720 nm, respectively. Based on an as-prepared few-layer HfGeTe optical switch and a Pr:LiYF4 gain medium, Q-switched visible lasers are also successfully achieved at 522, 640, and 720 nm. The minimum pulse widths of the green, red, and deep-red pulsed lasers are 150, 125.5, and 420 ns, respectively. Especially for the green and red pulsed laser, the obtained pulse width is smaller than those of the low-dimensional layered materials. Our work sheds light on the application potential of topological nodal-line semimetals in the generation of visible pulsed lasers.
visible pulsed laser topological nodal-line semimetals saturable absorption Q-switching Pr doping Chinese Optics Letters
2024, 22(3): 031601
1 四川大学电子信息学院图像信息研究所,四川成都 610064
2 中石油新疆油田分公司实验检测研究院,新疆克拉玛依 834000
石英和长石的识别对储集层研究具有重要意义。传统的矿物成分分析主要依靠人机交互式识别,工作量大且效率低,针对上述问题,提出一种利用岩石颗粒在正交偏光镜下的纹理特征进行识别的方法。首先用 Sobel算子提取样本图像的梯度信息,计算每个样本梯度图像的灰度共生矩阵的能量和相关性,利用能量和相关性为目标特征参数组建石英、长石特征参数样本库。应用人工神经网络 (ANN)分类方法进行训练,基于训练的结果,计算待识别颗粒的特征参数并分类。最后利用偏光序列图进行决策,得出最终识别结果。实验结果表明,此识别方法对石英和长石有较好的识别效果,识别率达 85%。
颗粒识别 正交偏光序列图像 Sobel变换 灰度共生矩阵 人工神经网络 particle identification orthogonal polarization sequence diagram Sobel transform Gray Level Co-occurrence Matrix Artificial Neural Network 太赫兹科学与电子信息学报
2015, 13(2): 285